Effortless Experience: Statistical Errors
Summary: The book The Effortless Experience presents impressive sounding statistics to show that the Customer Effort Score is a good predictor of customer loyalty. But should we believe the statistics? The authors use generous interpretations of some statistics and appear to perform some outright wrong statistical analyses. The mistakes cast doubt upon all the statistics in the study. This is the final review page of the book.
~ ~ ~
3. Flawed Application and Understanding of Statistics
First, let me state that I am not a statistician — despite what one of my former managers at Digital Equipment thinks. While I have more statistical training than most people, I am not a statistician. I know statisticians, and I’m not in their class. As I read the statistics Effortless presents, I got uncomfortable with the procedures and interpretations presented. So, I checked with two colleagues who are statisticians to make sure I was spot on.
Unfortunately, the folks who did this study don’t realize that they are not statisticians. Their statistical procedures are not the best ones to use in places. Their interpretation of statistics, is not accurate. And in one chart they manipulate some statistics in a completely erroneous way. It was this last point that showed me that they don’t have a true statistician on staff.
Just as with the description of the research processes, the description of their statistical procedures is sparse and scrambled. They throw out a few things, seemingly to impress. Granted, a detailed description of their statistical processes would be a snooze, but how about an appendix — or website — that presents what they did and the calculated statistics so that the educated reader could properly digest their research findings? At least tell us what statistical procedure you used to generate the impressive statistics. I had hoped that’s what I would find in the book, in contrast to the HBR article. I did not.
Below I describe some of the issues I saw with the statistical analysis, but I have included a couple of sections at the bottom of this page to provide a little more background information. One covers Statistical Analysis with Survey Data and the other Regression Equations with Survey Data.
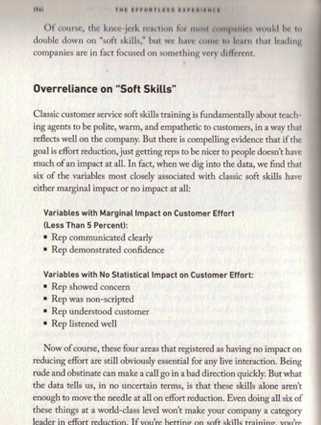
p-values in Analysis of Soft Skill Variables
Misunderstanding p-values. In the nearby image you will see a discussion about the importance of a call center agent’s soft skills to a customer’s positive feelings from page 96. Two factors have “Marginal Impact on Customer Effort (Less Than 5 Percent)” and four variables have “No Statistical Impact.” Once again, we have to guess at the analysis behind these statements. What’s 5% impact mean and where did it come from?
I am guessing the finding are based on p-values for each variable in a regression equation. The p-value tells us whether a variable — in this case, a survey question — has a statistically significant impact on the outcome variable. The lower the p-value, the higher the statistical significance. (Yes, that sounds counterintuitive. See the Dummies’ explanation of p-value.) If a variable has a p-value of “Less Than 5 Percent,” that does not mean it has a “Marginal Impact.” Quite the opposite! The lower the p-value the more important the variable is to have in the equation.
Regardless, the p-value does not tell use the “percent of impact,” which appears to be how the authors have interpreted the statistics. The lack of clarity here enhances the confusion, which emanates probably from the authors’ understanding of statistics.
Interpretation of Statistics. Let me return to that seminal Chapter 6. In talking about research done with CES 2.0, they state on page 159:
“First, we found that the new question wording was very strongly correlated to customer loyalty, explaining approximately 1/3 of customers repurchase intent, word-of-mouth, and willingness to consider new offerings… If one third of a customer’s loyalty intentions are driven by merely one service interaction, that is a pretty intimidating fact for most service organizations.” (emphasis added)
[For the statistically inclined, I believe this means the regression equation with only the CES question as an independent variable resulted in an R-squared of about 0.33.]First, let’s be generous and assume their statistical procedures and their statistics are accurate. The correct interpretation — what would get you close to an A on the statistics exam — is that one third of the variability in the dependent variable (loyalty measures) was explained by the independent variable (CES 2.0). Granted, that’s dorky, but it’s the right interpretation.
Second, as mentioned in a previous section on the research execution, the wording of the loyalty questions and the mental frame established for the respondents may mean they weren’t answering those questions based on “merely one service interaction.”
But without knowing anything about statistics, think about this claim. Let’s extend their argument. If the level of effort from “merely one service interaction” drives “33% of a customer’s loyalty intentions,” then would the effort from two service interactions drive 67% of loyalty intentions? Would 6 interactions’ effort drive 200% of loyalty intentions? Oops. That’s right. We can’t predict more than 100% of intended loyalty, can we?
Here’s the point. The customer loyalty questions — the dependent variable — measure intended customer loyalty at that point in time, not actual loyalty behavior. Each successive interaction, be it for product or service, will change intended loyalty, and actual loyalty behaviors will be affected by all these experiences and other factors. But their phrasing — “merely one service interaction” — implies a cumulative effect. Our ultimate loyalty decisions will be driven by perhaps scores of experiences with the product and related service. Each service interaction can’t explain one-third of future loyalty decision.
Now let’s step back and practice a little “face or conclusion validity” here, which is another way of saying “common sense.” Think about your service interactions with companies. How many times would you say that your level of effort from one service interaction determined a third of your actual loyalty behaviors with that company? Sure, we can all think of interactions that led you to leave a company or bond with it. But c’mon. Let’s get real. They’re saying on average the level of effort expended in one service interaction accounts for one-third of loyalty. The number is simply unbelievable and demonstrates again the weakness of the research procedures. These results should actually have made them step back and questioned their research, but instead, full steam ahead.
Recall that in a previous section we discussed how they directed respondents to select the service interaction to be described in the respondent’s answers. It likely led to a preponderance of negative service interactions being reported. The statistics described here may be the result of this possible flaw in how the researchers set the respondents’ mental frame.
Further, the “researchers” specifically only included service factors in their research model. What if product factors had been included? You can bet that “merely one service interaction” would not drive 33% of loyalty.
CES vs. Customer Satisfaction (CSAT) Impact on Loyalty. Also in Chapter 6 on page 159, the authors report:
When comparing this CES v2.0 to CSAT, we found the effort measured to be 12% more predictive of customer loyalty.
In the footnote on that page they explain how they determined that — and this is most detailed explanation of their statistical procedures in the entire book. And it’s relegated to a footnote.
We defined accuracy here by comparing the R-squared measure from two bivariate 0LS regression models with loyalty as the dependent variable.
Let’s put aside the interpretation of the statistics and the omission of “intended future.” My statistician colleagues tell me that this is not the best way to determine the impact of one independent variable versus another. The proper method is to put both in the model and then look at the change in the R-squared statistic when removing one variable.
Sense of False Precision — and Maybe Confusion About Data Properties. More generally, the presentation of the statistics as “12% more predictive” lends the impression that is an exact science. It is not. On page 162, they claim that
a 10% reduction in effort… would correspond to a significant 3.5% increase in customer loyalty.
I have no clear idea what “a 10% reduction in effort” means operationally. Is that reducing the survey scores on customer effort? If so, then they’re ascribing ratio properties when it’s debatable that the data even have interval properties. Stat geeks will understand that point — but it’s an important one. It would mean, then their understanding of basic statistical concepts is pitifully weak.
Regardless, that phrasing makes this sound like a laboratory experiment with precise measurements. Every survey has some amount of measurement and non-measurement error. (To see a brief discussion of these, see the Background section of my article on the impact of administration mode on survey scores, pages 2-6. It’s a bit academic dry, but readable.) As demonstrated in the above discussion, this research has loads of error in the data. The precision they imply is simply gross overstatement. But they’re trying to match the Reichheld hype machine with 1st decimal point precision.
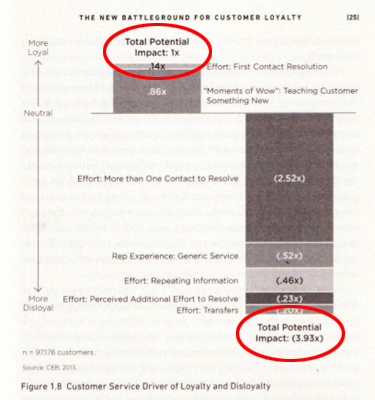
Figure 1.8: Implying Additive Properties for Regression Coefficients
Adding Regression Coefficients — An Absolute Mathematical No-No. The authors’ questionable understanding of statistics came into clear focus for me from Figures 1.5 and 1.8 in Chapter 1. I’ll focus on Figure 1.8 where they present the different factors they claim drive customer loyalty and disloyalty. But what are those numbers, and what are the “Xs”? They never say. We are left to guess at the analysis behind them.
They appear to me to be regression coefficients — beta coefficients — for each variable in the regression equation. (If I’m wrong I’ll apologize — AFTER the authors apologize for the paltry presentation.) The beta coefficients describe the relative impact of each independent variable (that is, survey question) upon the dependent variable, which in this case was some form of the loyalty questions. A little explanation would have been nice, but when your goal is to impress, why bother.
The point they are making is that more loyalty-negative factors entered the equation than positive, but again they don’t present us the critical statistics about the equation, which are the p-values for each variable (that is, survey question).
As outlined in the previous discussion, it appears the authors don’t understand the proper interpretation of p-values. So, did they properly apply p-values to build the regression model depicted in Figure 1.8? Maybe. Maybe not.
I also have to cycle back to the earlier discussion on the “mental frame” set for respondents. If it led respondents to describe a negative versus a positive service interaction, then naturally we would expect the model built from the data to focus on negative factors.
Let’s accept their model as valid. Look at the beta coefficients, which are described in greater detail in the section below. First Contact Resolution stands out as having the biggest impact on loyalty. What’s second most important? Wow Moments, which contradicts Effortless‘ proposition that we should stop delighting the customer.
Now notice what they included at the top or bottom of the two stacked bar columns, circled in the nearby image: Total Potential Impact upon loyalty, one for positive impact and one for negative impact. If I have surmised their analysis properly, they added the beta coefficients to arrive at a Total Potential Impact. That calculation is utter nonsense. (If it were an answer on a statistics exam, you’d be lucky to get 1 out of 10 points.) You can’t add coefficients from multiple terms in an equation. There is no logical interpretation for that derived number, and it shows a lack of understanding not of regression, but of basic 8th-grade algebra. (See the section below for more detail on regression equations and one possible explanation for what they did.)
If you don’t believe me, contact your college statistics professor and ask if it okay to add regression coefficients in a regression equation and what would be the interpretation of that summed number. Beware; you might be told to return for a refresher lest a belated grade change be entered on your transcript.
Additionally, the use of the stacked bar chart along with the label for the vertical axis implies that the coefficients are additive. They are not. The graph violates a key principle of Dr. Edward Tufte, the leading thinker of data visualization: Don’t imply a relationship that does not actually exist.
Perhaps the graphic designer went rogue and added that to the chart. But I doubt it. Perhaps the staff statistician was not on the proofreading committee. But I doubt it. This mistake, along with the apparent misunderstanding of p-values on page 96, casts a pall upon the statistical analysis in the whole book. Couple that with interpretations of some statistics that defy credulity and credibility as statisticians has been lost.
~ ~ ~
Conclusion: I find it stunning that numerous companies are buying the results from Effortless with apparently no probing questions or critical thinking about the methodological rigor of the research and analysis. The number and extent of shortcomings in the research presented in Effortless lead me to discount essentially all of their findings beyond findings generated from basic descriptive statistics.
However, I do agree in part with this statement (page 162):
[CES] is just a question. Make no mistake, it’s a powerful directional indicator, but that’s really all it is. CES is not the be-all, end-all, or silver bullet of customer service measurement, but rather part of a larger transactional loyalty measurement system. To really understand what’s going on in the service experience, you’ve got to look at it from multiple angles and through multiple lenses. You’ve got to collect a lot of data.
If only the authors had not presented CES as far more than a “powerful directional indicator” — and performed more credible research.
The Wizard of Oz implored, “Pay no attention to that man behind the curtain!” Always look behind the research curtain.
A Primer on Statistical Analysis with Survey Data
It appears that regression analysis was the primary statistical procedure. In a footnote on page 159, they mention doing “OLS regression.” OLS is Ordinary Least Squares, which they don’t spell out. Elsewhere, they present statistics that look like data from a regression model. However, doing advanced statistics with survey data is problematic because of the nature of the data set.
I believe their survey data are primarily “interval rating data,” data that we get from 1-to-5 or 1-to-10 scales. I saw “believe” because in places, e.g., pages 26 and 154, they describe their measures as binary, categorical measures – “loyal” versus “disloyal” and “high effort” versus “low effort” – not as scalar measures. If they converted scalar data to categorical, what were the cut-off points and on what basis?
The data are only truly interval if every respondent views the same consistent “unit of measurement” in the survey scales. Simply put, the cognitive distance from 1 to 2 is the same as 2 to 3, 9 to 10, etc. That’s a high hurdle that many academics say can never be achieved. More importantly here, regression analysis requires the data be interval. (Preferably, the data should have ratio properties, which we have if we’re measuring some physical quantity where there is a true zero on the scale, such as time, length, height. With ratio data we can multiply, divide, and take percentages.)
The authors never mention the lack of interval properties as a potential shortcoming to their analysis. Did they test for the linearity of the data? If the data aren’t linear, which they argue in Figure 1.3, then other types of regression than OLS are the more appropriate procedure. Did they consider using Chi Square analysis, which only requires ordinal data properties? That’s the safe approach with these type of questions.
Also, the study has multiple measures of loyalty. How did they handle this in their model? My guess — and I have to guess — is that they simply added the scores for those questions from each respondent to create one dependent variable. This process means that each loyalty measure has equal importance in describing loyalty. Is that reasonable? How did they determine that those questions describe loyal behavior? These details matter. That’s where the devil resides.
A Primer on Regression Equations with Survey Data
For many readers, discussing regression equations is a good sleep inducer, so I’m putting it at the bottom of the article. Get a cup of coffee. The basic regression equation is:
y = a + b 1×1 + b 2×2 + b 3×2 + b 4×4 + b 5×5+…+ error
Those funny Bs are “Beta coefficients” and the Xs each represent a different variable or survey question. Let’s get away from Greek letters and symbols. It’s saying
Outcome or Dependent Variable = ¦unction of (Causal or Independent Variables)
That is, loyalty is driven by several factors in a service interaction. Specifically, in this case, the authors are saying:
Customer Loyalty = Y intercept
+ 0.14(1st Contact Resolution)
+ 0.86(Wow Moments)
– 2.52(More Than 1 Contact to Resolve)
– 0.52(Generic Service)
– 0.46(Repeating Information)
– 0.23(Perceived Additional Effort to Resolve)
– 0.20(Transfers)
Each of those phrases (e.g., Customer Loyalty), is a variable in the equation, which in this case are the survey questions. The number that would be plugged into the above equation is the mean score for each survey question.
To determine whether the variable (survey question) should be in the model, we examine the p-values for each variable, which tells us whether that survey question is statistically significant. The authors don’t share with us these statistics, so we have to assume that they have built the model correctly.
Here’s the interpretation of Beta Coefficients — in terms of this equation. The coefficient shows how much the mean survey score for customer loyalty would increase or decrease if the mean survey score for one of the causal factors changed by 1. So, if the mean survey score for 1st Contact Resolution increased by 1, then we’d expect the mean survey score for Customer Loyalty would increase by 0.14.
Think back to your high school algebra. The only circumstance in which you could add the Beta coefficients is if x1=x2=x3 and so on. That is certainly not the case here since each x represents the mean score of each survey question in the model. However, in the chart they do not differentiate the Xs, which is why it’s possible the graphic designer decided to add the coefficients as a cool feature. (I’m trying to be generous here and blame the egregious error on the graphic designer.)
Adding the coefficients is mathematically wrong and has no legitimate interpretation. But it’s good for hype. This is a startling mistake for trained researchers to make and should make us question all the statistical analysis.
There is one possible explanation for their interpretation of the statistics. It is possible that they converted the variables measured with interval-rating survey scales into categorical variables. For example, a question that measured whether the agent delivered “generic service” using a 1-to-7 scale could be converted into a binary yes-no variable that the agent did or did not deliver generic service. In that case, they may have used dummy variables in the regression model where the coefficients shift the y-intercept of the regression line rather than change the line’s slope. Adding the coefficients in this case would be more acceptable, but note how they present the numbers with a trailing “x”, which implies multiplication. That’s why I don’t think they used dummy variables. If they did, then how did they determine the threshold points for converting interval measures to categorical measures. Again, some explanation would be so nice.
See the related articles:
“The Effortless Experience” Book Review
1. Weak & Flawed Research Model
2. Questionnaire Design & Survey Administration Issues